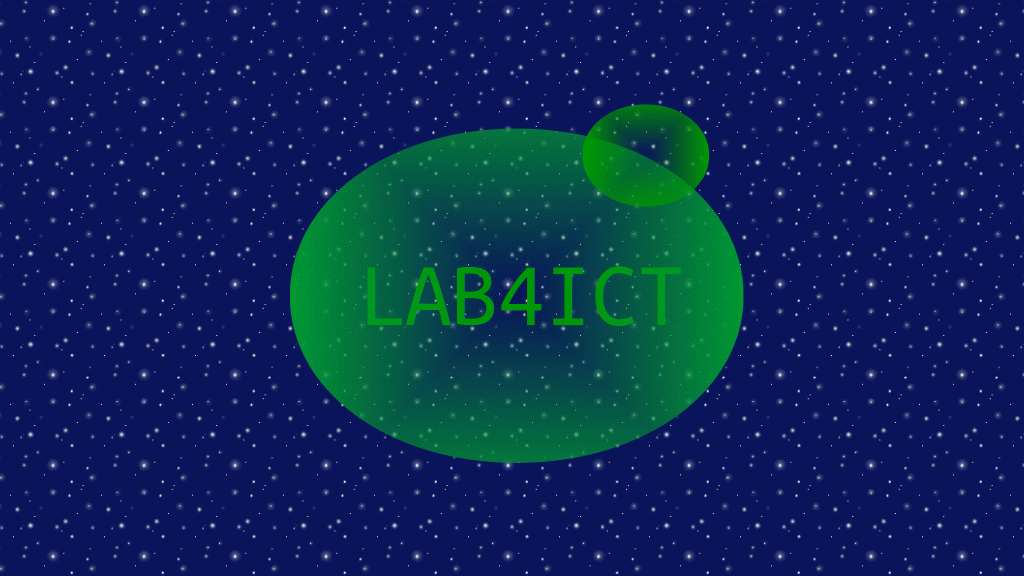
Ubuntu 16.04 LTS Desktopに、CUDAによるTensorFlowのディープラーニング環境を構築します。本校執筆時では、最新のCUDA 9.2を使用するためにはTensorFlowのコンパイルが必要なため、環境構築が容易なCUDA 9.0を使用します。もう一つ重要なポイントが、AVX機能を持つCPU(Sandy bridge以降のCore iシリーズ)を使用することです。AVX機能がないCPU(特にCeleron、Pentium)を使用する場合は、TensorFlowをソースからコンパイルするか、バージョン1.5以前を使用する必要があります。旧型のCPUを使用する場合は、AVX機能があるCPUであるかよく確認する必要があります。
記事の目次
使用した環境
- Core i5-7500
- GeForce GTX 1050 Ti
- Ubuntu 16.04 LTS Desktop (16.04.5)
- CUDA Toolkit 9.0
- cuDNN 7.2
- Anaconda 5.2 (Python 3.6)
- TensorFlow (GPU)
CUDA Toolkit 9.0のインストール
CUDA Toolkit 9.0をインストールします。
CUDA Toolkit 9.0のダウンロード
CUDA 9.0のインストール用モジュールを、Nvidiaのホームページ「CUDA Toolkit 9.0 Download」から以下を選択して、ダウンロードします。
- Operating System : Linux
- Architecture : x86_64
- Distribution : Ubuntu
- Version : 16.04
- Installer Type : dev [network]
CUDA Toolkit 9.0のインストールの実行
以下のコマンドを実行して、CUDA Toolkit 9.0をインストールします。デバイスドライバーも同時にインストールされます。
$ cd $HOME/Downloads $ sudo dpkg -i cuda-repo-ubuntu1604_9.0.176-1_amd64.deb $ sudo apt-key adv --fetch-keys http://developer.download.nvidia.com/compute/cuda/repos/ubuntu1604/x86_64/7fa2af80.pub $ sudo apt-get update $ sudo apt-get install cuda-9-0
インストールが終了したら、ドライバーを有効にするため、OSを再起動します。
CUDA Toolkit 9.0のインストールの検証
CUDA Toolkit 9.0のインストールの検証を行います。nvidia-smiコマンドを実行して、GPUの情報が表示されることを確認します。ドライバーのバージョンも確認できます。
nvidia-smi Fri Sep 14 23:25:47 2018 +-----------------------------------------------------------------------------+ | NVIDIA-SMI 396.44 Driver Version: 396.44 | |-------------------------------+----------------------+----------------------+ | GPU Name Persistence-M| Bus-Id Disp.A | Volatile Uncorr. ECC | | Fan Temp Perf Pwr:Usage/Cap| Memory-Usage | GPU-Util Compute M. | |===============================+======================+======================| | 0 GeForce GTX 105... Off | 00000000:01:00.0 Off | N/A | | 35% 34C P0 N/A / 75W | 184MiB / 4040MiB | 0% Default | +-------------------------------+----------------------+----------------------+ +-----------------------------------------------------------------------------+ | Processes: GPU Memory | | GPU PID Type Process name Usage | |=============================================================================| | 0 1202 G /usr/lib/xorg/Xorg 28MiB | | 0 1420 G /usr/lib/xorg/Xorg 57MiB | | 0 1755 G /usr/bin/gnome-shell 66MiB | +-----------------------------------------------------------------------------+
cuDNN 7.2のインストール
デープラーニング用のライブラリcuDNN 7.2をインストールします。
cuDNN 7.2のダウンロード
cuDNN 7.2のインストール用モジュールを、Nvidiaのホームページ「NVIDIA cuDNN」から「Download cuDNN」ボタンをクリックして画面遷移し、ユーザ登録を行い、ダウンロードページにログインします。CUDA 9.0用のページに遷移して、以下を選択して、ダウンロードします。
- cuDNN v7.2.1 Runtime Library for Ubuntu16.04 (Deb)
- cuDNN v7.2.1 Developer Library for Ubuntu16.04 (Deb)
- cuDNN v7.2.1 Code Samples and User Guide for Ubuntu16.04 (Deb)
cuDNN 7.2のインストールの実行
以下のコマンドを実行して、cuDNN 7.2をインストールします。
$ cd $HOME/Downloads $ sudo dpkg -i libcudnn7_7.2.1.38-1+cuda9.0_amd64.deb $ sudo dpkg -i libcudnn7-dev_7.2.1.38-1+cuda9.0_amd64.deb $ sudo dpkg -i libcudnn7-doc_7.2.1.38-1+cuda9.0_amd64.deb
cuDNN 7.2のインストールの検証
cuDNN 7.2のインストールの検証を行います。最後に、「Test passed!」と表示されることを確認します。
$ cp -r /usr/src/cudnn_samples_v7/ $HOME $ cd $HOME/cudnn_samples_v7/mnistCUDNN $ make clean && make $ ./mnistCUDNN
Anaconda 5.2のインストール
python環境を構築するため、Anacondaをインストールします。
Anaconda 5.2のダウンロード
Anacondaのダウンロードページ「Download Anaconda Distribution」から、python 3.6バージョンのanacondaをダウンロードします。
Anaconda 5.2のインストールの実行
以下のコマンドで、Anaconda 5.2をインストールします。途中、ライセンスへの同意、PATH変数への登録、VSCodeの導入を聞かれますが、以下の例では、すべて"yes"でインストールを行います。
$ bash ~/Downloads/Anaconda3-5.2.0-Linux-x86_64.sh ... In order to continue the installation process, please review the license agreement. Please, press ENTER to continue >>> ... Do you accept the license terms? [yes|no] [no] >>> yes ...Do you wish the installer to prepend the Anaconda3 install location to PATH in your /home/sysadm001/.bashrc ? [yes|no] [no] >>> yes... Do you wish to proceed with the installation of Microsoft VSCode? [yes|no] >>> yes ... VSCode successfully installed in /usr/share/code !
Anaconda 5.2のインストールの検証
anacondaのナビゲータが起動することを確認します。
$ source ~/.bashrc $ anaconda-navigator
TensorFlowのインストール
TensorFlowをインストールします。
TensorFlowのインストールの実行
pythonの環境を確認します。
$ python -V Python 3.6.5 :: Anaconda, Inc. $ pip -V pip 10.0.1 from /home/sysadm001/anaconda3/lib/python3.6/site-packages/pip (python 3.6)
以下のコマンドで、GPU対応のTensorFlowをインストールします。
$ pip install --upgrade --user tensorflow-gpu
TensorFlowインストールの検証
TensorFlowのサンプルコードを実行し、「b'Hello, TensorFlow!'」の文字列が出力され、TensorFlowが使用できることを確認します。以下の例は、CPUが「Core i5-7500」の環境を使用したため、AVX2 FMAを使用するようにコンパイルされていないとのメッセージが出力されています。その他、GPUの情報も出力されました。
$ python Python 3.6.5 |Anaconda, Inc.| (default, Apr 29 2018, 16:14:56) [GCC 7.2.0] on linux Type "help", "copyright", "credits" or "license" for more information. >>> import tensorflow as tf /home/sysadm001/anaconda3/lib/python3.6/site-packages/h5py/__init__.py:36: FutureWarning: Conversion of the second argument of issubdtype from `float` to `np.floating` is deprecated. In future, it will be treated as `np.float64 == np.dtype(float).type`. from ._conv import register_converters as _register_converters >>> hello = tf.constant('Hello, TensorFlow!') >>> sess = tf.Session() 2018-09-15 16:46:26.839201: I tensorflow/core/platform/cpu_feature_guard.cc:141] Your CPU supports instructions that this TensorFlow binary was not compiled to use: AVX2 FMA 2018-09-15 16:46:27.377131: I tensorflow/stream_executor/cuda/cuda_gpu_executor.cc:897] successful NUMA node read from SysFS had negative value (-1), but there must be at least one NUMA node, so returning NUMA node zero 2018-09-15 16:46:27.378222: I tensorflow/core/common_runtime/gpu/gpu_device.cc:1405] Found device 0 with properties: name: GeForce GTX 1050 Ti major: 6 minor: 1 memoryClockRate(GHz): 1.455 pciBusID: 0000:01:00.0 totalMemory: 3.95GiB freeMemory: 3.64GiB 2018-09-15 16:46:27.378276: I tensorflow/core/common_runtime/gpu/gpu_device.cc:1484] Adding visible gpu devices: 0 2018-09-15 16:46:36.710863: I tensorflow/core/common_runtime/gpu/gpu_device.cc:965] Device interconnect StreamExecutor with strength 1 edge matrix: 2018-09-15 16:46:36.710945: I tensorflow/core/common_runtime/gpu/gpu_device.cc:971] 0 2018-09-15 16:46:36.710966: I tensorflow/core/common_runtime/gpu/gpu_device.cc:984] 0: N 2018-09-15 16:46:36.716621: I tensorflow/core/common_runtime/gpu/gpu_device.cc:1097] Created TensorFlow device (/job:localhost/replica:0/task:0/device:GPU:0 with 3371 MB memory) -> physical GPU (device: 0, name: GeForce GTX 1050 Ti, pci bus id: 0000:01:00.0, compute capability: 6.1) >>> print(sess.run(hello)) b'Hello, TensorFlow!' >>>
GPUを使用しない場合
GPUを使用せず、CPUのみでディープラーニングを行う場合は、本稿の「Anaconda 5.2のインストール」から開始し、TensorFlowのインストールは、以下のCPUなしのコマンドでインストールを行います。
pip install --upgrade --user tensorflow
おわりに
本稿では、TensorFlowをインストールしました。インストール環境がサポートされている環境であれば比較的容易にインストールを行うことができます。ただし、TensorFlowがまだCUDA 9.2に対応していないことや、AVX機能がないCPUを使用する場合はソースからのコンパイルを行うか旧バージョンを使用する必要があることに注意します。
参考情報
- CUDA Toolkit Archive
- Nvidia cuDNN
- Nvidia DEEP LEARNING SDK DOCUMENTATION
- Download Anaconda Distribution
- Anaconda Installing on Linux
- Install Tensor Flow
関連記事
関連書籍